To Issue 127
Citation: Benassi A, Cottini C, “Numerical Simulations for Inhalation Product Development: Achievements and Current Limitations”. ONdrugDelivery, Issue 127 (Nov 2021), pp 68–75.
Andrea Benassi and Ciro Cottini discuss the current state of CFD and DEM computational modelling, how it is used to improve inhaler design and manufacturing, and what the current limitations of this technology are, as well as how those limitations may be overcome.
“Powder-fluid interaction is an intrinsically complex and non-linear phenomenon that is still both difficult to accurately model and poorly understood at the fundamental level.”
The role played by physical and engineering simulation techniques in the design of pharmaceutical products, and related manufacturing processes, has, undoubtedly, grown rapidly in recent years. The increasing number of technical publications in scientific literature (Figure 1), the appearance of modelling and simulation services in the portfolio of many contract research and manufacturing organisations working with pharma companies, and the opening of new funding opportunities from regulatory agencies to explore the reliability of such simulations for pharma and medical applications1 are all testament to this growth. Many application areas can be identified in the context of inhalation products, amongst which the most notable are:
- Manufacturing process development
- Inhaler design
- Modelling of drug delivery to the lungs.
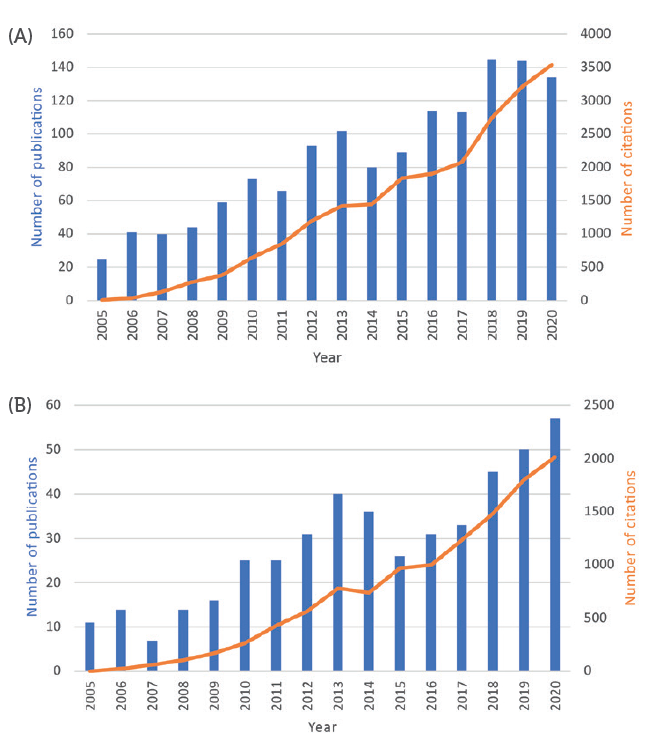
Figure 1: Number of published papers per year, and their citations, with topics related to CFD/DEM simulation of pharmaceutical processes (A) and CFD/DEM applied to inhaler design and drug deposition in the lungs (B).
A trait common to most of these applications is the presence of pharmaceutical powders interacting with fluids (liquids or gasses). This occurs both during the powder manufacturing process and the use of the device by the patient, some specific examples being:
- A drug substance powder crystalising and precipitating in a liquid solvent in a synthesis reactor
- A drug product powder dose entrained and aerosolised by a swirling air flow in a dry powder inhaler
- Airborne drug substance particles travelling down the patient’s tracheo-bronchial tree.
Powder-fluid interaction is an intrinsically complex and non-linear phenomenon that is still both difficult to accurately model and poorly understood at the fundamental level. Predictions based on simple rules of thumb or empirical formulae are impossible, especially in complex geometries such as industrial machinery, inhalers or human airways. In most cases, a direct measure of powder and fluid behaviour is difficult or impossible due to:
- Harsh environments, such as hot corrosive solvents in reactors or abrasive conditions in powder mills
- Limitations imposed by quality and safety protocols in manufacturing for human-use productions
- Difficulty in measuring fast phenomena occurring in extremely limited volumes, such as drug dose aerosolisation inside an inhaler camber
- Absence of easy access to the product, such as in sealed bins during mixing
- Lack of suitable devices for taking measurements.
The success of simulation-based design stems precisely from these limitations – being able to reproduce the behaviour of products during manufacturing and administration in silico (i.e. using computer simulations) allows for a better understanding of the underlying mechanisms governing these interations and a more conscious approach to product design and optimisation, eventually leading to performance and quality enhancements.
Simulations can also be fed with data measured directly from patients, such as inhalation profiles recorded via spirometry or 3D lung morphologies acquired with CT or MRI scanners, becoming a formidable tool in a patient-centric drug product design approach. To take the principle further, study and optimisation of inhaler performance could be conducted for specific populations of patients with peculiar lung morphologies and/or inspiratory profiles as a consequence of different degrees of disease severity or comorbidities.
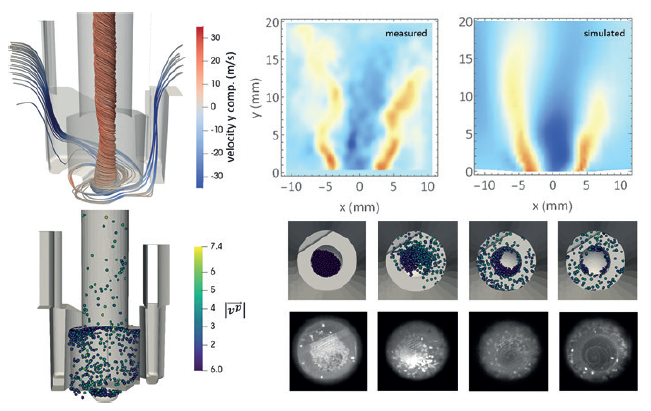
Figure 2: Coupled CFD-DEM has been used to model the emission of particles from a dry powder inhaler during inspiration.2
SIMULATION TECHNIQUES AND THEIR VALIDATION
Despite the great variety of possible applications, the simulation methods employed are always the same. Computational fluid dynamics (CFD) is used to simulate the fluid behaviour, based on the solutions of Navier-Stokes equations, while discrete element methods (DEM) are used to reproduce a powder flow by solving the Newton’s equation of motion for each individual particle composing it.
When describing a powder entrained and interacting with a fluid, these two simulation techniques must be coupled together (Figure 2), with different coupling conditions used depending on the level of dispersion of the powder particles.3 In the case of very diluted powder suspensions, a one way coupling is typically adopted. In this approximation, the fluid drags along the particles, but the energy lost in the process is considered negligible – thus the fluid is not perturbed at all by the powder’s presence. When the powder concentration is high, the energy and momentum exchanged with the fluid are no longer negligible and therefore a two-way coupling is necessary. In this case, the fluid accelerates the powder mass and, in so doing, decelerates. In one-way coupling conditions the particles are so diluted that they do not feel each other, however, as the particle density increases they perceive other particles by the perturbations they leave in the fluid (wakes) – an effect typically referred to as three-way coupling. Finally, when the powder density is significantly high, particle-particle collisions and inter-particle interactions start to become relevant. Including them means running a fully coupled, or four-way coupling, simulation.
Both the simulation methods make use of different approximations and assumptions, and can also depend on certain model parameters not known a priori. The level of realism and the reliability of simulations must therefore be evaluated by comparing their outcomes with experimental data – a procedure known as simulation validation. On the other hand, a model calibration is necessary when a value must be set for a parameter that is not known a priori and not easily experimentally measurable. This is typically the case for certain DEM parameters determining the interparticle interaction, such as the elasticity of the collisions or the friction forces between particles. In this case many simulations are run varying that free parameter until a good match is found with experimental data. Clearly, there must be only one value (or a narrow range of values) for a given parameter where the simulation result and experimental data match. However, verifying this for increasing numbers of free parameters becomes very challenging.
As mentioned prior, acquiring experimental data is neither easy nor always possible in the regions of interest of a manufacturing facility, piece of equipment or drug delivery device, thus model validation/calibration is usually performed on simplified geometries or for small-scale laboratory prototypes. In practice, this means using simplified working conditions that are still representative of the real processes, but where data acquisition is much easier. In some other cases it is not possible to measure what is going on in a region of interest, but it is easy to measure what enters and exits from that region. Thus, some validations and calibrations are performed by matching the physical quantities and profiles measured at inlets and outlets of a system to those of a simulation.
MANUFACTURING PROCESS DEVELOPMENT
Among all application areas, manufacturing process development is the widest, encompassing particle engineering applications such as spry-drying and milling,4-6 design of manufacturing unit operations such as dry powder mixing, tableting and spray-coating,7 and design of equipment and facilities such as freeze dryers or bio-reactors.8-11 Covering all these aspects is beyond the scope of this brief perspective and many details are intimately connected to each specific application. Most of the works on modelling and simulation are not even found in the pharmaceutical technology literature – they belong more generally to the chemical engineering and powder technology realm.
In cases where the scale of the problem or the total mass/volume of product involved is large, surface effects and other details are negligible, so a satisfactory description of the phenomena can be achieved by simulating only the average properties of the fluids and powders. The fluid turbulence and its interaction with single powder particles can be neglected, as well as the correct shape of the particles – usually assumed to be spherical. For such cases, standard commercial software already provides all the necessary features and the validation of the simulations is straightforward.
In other cases, where a specific feature is extremely relevant but many others can be neglected, such as the spray-coating of tablets, it is necessary to model the real tablet shape with a high degree of accuracy while the presence of air in the tumbler can be completely neglected. This means that, under such circumstances, it is possible to work with DEM simulations alone.
Finally, applications exist that challenge even state-of-the-art software. For example, in jet mills, particle size reduction is achieved through a supersonic gas accelerating a large mass of powder in order to promote particle-particle collisions and breakage. Simulating such phenomena requires specific CFD algorithms able to treat transonic and supersonic gas flows, while fully coupling them with DEM simulations of a large mass of powder (Figure 3). Such a requirement is beyond the capabilities of most commercially available software. Furthermore, on top of that, a good description of particle fragmentation is necessary and specific models coming from fracture mechanics must be implemented in the DEM software.13,14 To date, the combination of all these elements is still missing, and as such it is not possible to simulate the jet milling process to a satisfactory level.
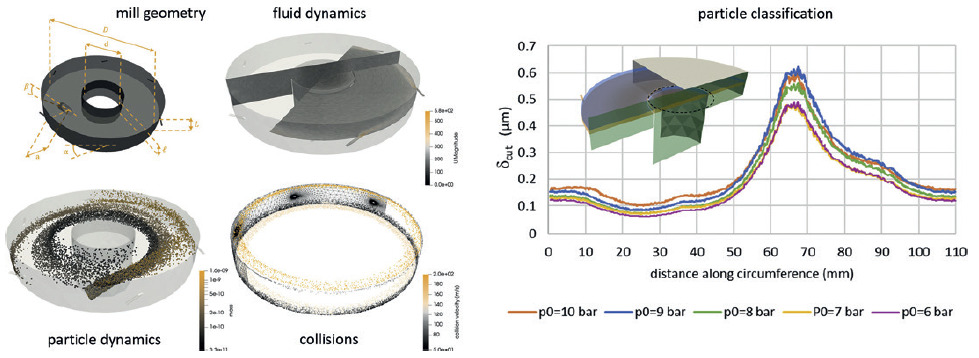
Figure 3: While CFD-DEM analysis can be used to gain insights into the mechanics of jet milling, current technology is yet to produce a satisfactory simulation the covers all elements of the process simultaneously.12
To conclude, it must be noted that it is only on rare occasions that it is possible to build digital twins for pharmaceutical processes. The need to account for the properties of both fluids and powders makes the simulations complex, usually requiring dedicated computational resources and taking many hours, or even days, to obtain the numerical results and process them. Thus, although very useful during the design phase of processes and products, coupled CFD-DEM simulations are rarely able to give real-time information during batch manufacturing.
“Although very useful during the design phase of processes and products, coupled CFD-DEM simulations are rarely able to give real- time information during batch manufacturing.”
DESIGN OF INHALERS
The methods for collecting certain data from outside inhaler devices are well established:
- Air flow rate and pressure drop profile can be easily recorded at the inlets and outlets of inhalers
- Particle and droplet emission time and size distribution can be measured optically at the device outlet15-17
- Temperature and the shape of aerosol plumes can be imaged with fast thermo-cameras.18,19
Measuring what happens inside devices is definitely more complex. In most cases, X-ray-based methods are used to image fast phenomena, such as the flash boiling of a propellant in pressurised metered dose inhalers (pMDIs)20 or the initial lift of carrier particles in a carrier-based dry powder inhaler (DPI),21 but quantitative data are rare. In this sense, simulations can be extremely helpful – after validating/calibrating them with the data available for both fluids and aerosol particles at the outlet, they offer a complete insight into what happens inside inhalers.
In DPIs, for instance, carrier particle collisions as well as API agglomerate breakage by air turbulence are known to enhance inhalation performances, in particular the fine particle fraction. Such phenomena can be easily quantified and studied with a validated CFD-DEM model.22-25 In silico geometry optimisation studies can be performed on inhalers to maximise both such phenomena and the conditions that lead to API aerosolisation and deagglomeration.26,27 Finally, inhalation profiles of different patient populations can be used as boundary conditions for the simulations to see how an inhaler responds to weak inspiratory acts, or how ineffective it becomes in misuse conditions.
This kind of information can be used during inhaler design to improve patient compliance and to customise it for specific patient needs, achieving high performance even in the case of weak flow conditions. For DPIs, when the drug dose is initially concentrated at rest in a cup/pocket or in a capsule, fully-coupled simulations are fundamental to correctly capture an accurate model of the aerosolisation process.22
“The possibility of simulating the deposition of aerosol particles in the respiratory system reliably is now concretising, thanks to the recent and substantial progresses in in vivo real-time 3D functional imaging.”
Improving On the State of the Art
Due to intrinsic limitations of the DEM technique, it is very difficult to simultaneously simulate both the carrier particles and the fine API particles in carrier-based DPIs. For a qualitative understanding of the API detachment and disaggregation mechanisms, simulations can be performed with the carrier surface covered with smaller particles that still have a diameter 50–100 times larger than real API particles – this means that the total number of API particles in the simulated dose will be orders of magnitude smaller than in reality. The tough part is that API-carrier adhesion forces, elasticity and frictional coefficient must be set in the DEM simulation through a complex calibration procedure.28
In aiming to achieve quantitative and predictive simulations of DPIs, an implicit approach has been suggested and successfully employed where only the carrier particles are simulated, while fine API particles are treated as a continuum phase diluted in air.29 The concentration of API particles is increased close to a carrier particle depending on its relative velocity with respect to air and any time it experiences collisions. This approach requires a huge effort in the parametrisation of the API release from carrier particles, but allows for the simulation of a realistic concentration of API inside the air.
No matter how fast, and thus turbulent, the air flow is in the inhaler, close to the inner walls its velocity must drop to zero, which creates a narrow region, called the boundary layer, with strong air velocity gradients. With the typical flow rates of DPIs inhalers, the thickness of this layer is estimated to be few hundreds microns – comparable to the size of the carrier particles and much larger than that of the API particles.30,31 This means that both carrier and fine API particles sitting at the bottom of an inhaler, as well as API particles on the surface of carrier particles, feel the presence of such a layer. Corrections of particle-air forces in the vicinity of walls, to account for the existence of this boundary layer, are not implemented in most of the available software.
Particles of lesser size (and thus reduced inertia), such as the API ones, are strongly influenced in their motion by turbulence in the air flow entraining them. Exactly as random thermal fluctuations generate a stochastic Brownian motion for nano-sized particles, random air velocity fluctuations (turbulence) generate a stochastic trajectory for those particles small enough to be entrained in the turbulent eddies. To reduce the computational cost, in most industrial CFD simulations the presence of turbulence is included without explicit simulation of the velocity fluctuations, not at least at all the scales at which these fluctuations occur. Thus, when dealing with small particle dispersion, particle-turbulence interaction must be re-introduced with an ad-hoc stochastic term.30,32 These particle turbulence effective interaction models are not always implemented in most of the available software and, when present, their general-purpose implementation is questionable.33,34
Simulating the behaviour of drug products during the actuation of a pMDI is much more complex, owing to the occurrence of the propellent flash-boiling phase transition – the phase change and latent heat exchange require a complex treatment of the thermodynamics of the fluid mixture. Many models for the nucleation of gas bubbles have never been tested in this context, and in general they are not even implemented in most of the open source or commercially available software. For these reasons most of the models available to predict drug product behaviour inside a pMDI actuator are based on phenomenological zero-dimensional models – time dependent equations describing the thermodynamic properties without any spatial resolution.35,36 It is only recently that some progress and a first implementation has been made available.37
“Scientific software development requires great effort – one that pharma companies are not well suited to undertake – however, commercial software companies are not always keen to implement cutting-edge algorithms, still under debate in the academic literature, that are of limited interest among their broader userbase.”
DRUG DELIVERY TO THE LUNGS
The possibility of simulating the deposition of aerosol particles in the respiratory system reliably is now concretising, thanks to the recent and substantial progresses in in vivo real-time 3D functional imaging.38,39 These techniques not only provide realistic models of 3D lung geometry to be used with CFD, but they also allow investigators to monitor the API concentration inside the patient lungs, thus providing data to validate the simulated deposition. In the extra-thoracic airways and nose, where air flow is dominated by turbulence, its explicit inclusion in the CFD simulations has proven to be necessary to achieve realistic results in the description of particle deposition. In other cases, simulations are validated against data collected on casts realised from realistic 3D models constructed using 3D printing or moulding.40,41 The advantage of these experiments is that that air flow conditions are much more controllable and reproducible, as well as that, besides deposition data, air velocity maps can be recorded (using particle image velocimetry) in mouth, throat and upper airway generations.
Aerosol particles are assumed to be already highly diluted while entering the patient mouth, thus a one-way coupling is generally used. The deposition in the intrathoracic generations is entirely dominated by inertia for particles above 10 microns, while Brownian diffusion plays a major role for particles below 0.5 microns. The intermediate range between 10 and 0.5 microns – the most interesting one from the drug delivery point of view – is the most complex one to simulate, where the effect of the weak turbulence, surviving from the upper airways, might contribute to deposition comparable to sedimentation and to inertia-driven impactions from the secondary flow. To the best of our knowledge, the contribution of the different deposition mechanisms for this intermediate range of particles in the conductive intra-thoracic airways remains unclear and requires further investigation.
However, as the diameter of the airways decreases, it drops below the resolution of the scanner around the sixth or seventh airway generations, which prevents an imaging-based reconstruction of the lung. Mathematical models are thus used to continue the lung reconstruction of the lower functional generations up to the alveolar sacs.42-45 Whole-lung models use multi-scale CFD simulations of multiple branching paths to complete the deposition statistics in the lower airways.46
Alternatively, statistical approaches can be used to simulate the deposition in the lower airway. Here the laminar (simpler) nature of the air flow allows the calculation of analytical expressions for the deposition probabilities.30,47 However, the uncertainties in the mathematical models used to generate the bifurcations and the absence of spatially resolved deposition data for the deeper airways reduce the reliability of deep airway simulation. Most of the explicit CFD-DEM simulations found in literature are thus limited to the upper airways only, which, when compared with deposition data, usually demonstrate discrepancies of less than 10%.46,48
There is, of course, room for improvement – small particles (5 to 0.5 microns) are known to accumulate and travel in the boundary layer, but many simulations approach this region using wall functions. In cases where turbulence is explicitly simulated, typically large eddy simulations, a cut-off is usually adopted, neglecting the small eddies that populate the region close to device walls. The implications of such approximations in the particle-fluid interaction, and thus on particle depositions, are still unclear and poorly debated in the current literature. Efforts are needed to improve the description of the lung model, for instance allowing the 3D lobes to change their volume and enabling certain anatomical features, such as the glottis, to move during the inspiratory act.49 Finally, in pursuit of a predictive tool that is able to resolve the spatial deposition of API particles in every airway generation, attempts are ongoing to couple it with pharmacokinetic and pharmacodynamic models to improve our understanding and predictions for bioavailability and systemic exposition.50
CONCLUSION
CFD and DEM simulations are proving to be valuable tools for product and process design, offering new insights into poorly understood phenomena and enabling new predictive capabilities. Best practices and reference standards have been recently proposed for specific CFD-DEM simulations of inhalers51 and human lung deposition.52 Indeed, there is still plenty of room for improvement, as well as new applications and challenges for the current state of the art of technologies and algorithms.
However, to fully exploit the potential of modelling and simulation, a cultural change must take place in the pharmaceutical manufacturing sector, a change that was started with the introduction of quality by design principles, the digital revolution and the Industry 4.0 philosophy, but that has not yet been completed. To master the complexity of CFD and DEM simulations, competences in physics, computational engineering and scientific high-performance computing are needed. However, physicists, engineers and information technicians are rarely found among the ranks of research professionals currently employed in drug product design.
Most of the software for CFD and DEM simulations is developed to be general purpose and lacks certain features necessary for specific pharma applications. Scientific software development requires great effort – one that pharma companies are not well suited to undertake – however, commercial software companies are not always keen to implement cutting-edge algorithms, still under debate in the academic literature, that are of limited interest among their broader userbase. A possible solution is the adoption of open-source software, for which customisation and implementation of these cutting-edge algorithms could be achieved through partnerships with academic research groups and scientific computing centres. With this in mind, the culture in the industry should evolve from a contract/consultancy driven collaboration to an open innovation approach.
REFERENCES
- “FY 2020 GDUFA Science and Research Report”. US FDA, Jan 2021.
- Ponzini R et al, “Coupled CFD-DEM Model for Dry Powder Inhalers Simulation: Validation and Sensitivity Analysis for the Main Model Parameters”. Powder Technol, Jun 2021, Vol 385, pp 199–226.
- Norouzi HR et al, “Coupled CFDDEM Modeling: Formulation, Implementation and Application to Multiphase Flows”. Wiley, 2016.
- Longest PW et al, “Computational Fluid Dynamics (CFD) Simulations of Spray Drying: Linking Drying Parameters with Experimental Aerosolization Performance”. Pharm Res, May 2020, Vol 37, Article 101.
- Bnà S et al, “Investigation of Particle Dynamics and Classification Mechanism in a Spiral Jet Mill Through Computational Fluid Dynamics and Discrete Element Methods”. Powder Technol, Mar 2020, Vol 364, pp 746–773.
- Keshani S, “Spray Drying: An Overview on Wall Deposition, Process and Modelling”. J Food Eng, Feb 2015, Vol 146, pp 152–162.
- “Predictive Modeling of Pharmaceutical Unit Operations” (Pandey P, Bharadwaj, eds). Woodhead Publishing, 2017.
- Barresi AA, Marchisio DL, “Computational Fluid Dynamics data for improving freeze-dryers design”. Data Brief, Aug 2018, Vol 19, pp 1181–1213.
- Langrish T et al, “Using CFD Simulations to Guide the Development of a New Spray Dryer Design”. Processes, Aug 2020, Vol 8(8), Article 932.
- Li X et al, “Development of a Computational Fluid Dynamics Model for Scaling-up Ambr Bioreactors”. Biotechnol Bioproc, Dec 2018, Vol 23, pp 710–725.
- Rathore AS, Shekhawat LK, Loomba V, “Computational Fluid Dynamics for Bioreactor Design” in “Bioreactors: Design, Operation and Novel Applications” (Mandenius CF, ed). Wiley, 2016, pp 295–322.
- Bnà S et al, “Investigation of Particle Dynamics and Classification Mechanism in a Spiral Jet Mill Through Computational Fluid Dynamics and Discrete Element Methods”. Powder Technol, Mar 2020, Vol 364, pp 746–773.
- Meier M et al, “Influence of Mechanical Properties on Impact Fracture: Prediction of the Milling Behaviour of Pharmaceutical Powders by Nanoindentation”. Powder Technol, Jan 2009, Vol 188(3), pp 301–313.
- Bruchmüller J et al, “Modelling Discrete Fragmentation of Brittle Particles”. Powder Technol, Apr 2011, Vol 208(3), pp 731–739.
- Pasquali I, “Optical Diagnostics Study of Air Flow and Powder Fluidisation In Nexthaler® – Part I: Studies with Lactose Placebo Formulation”. Int J Pharm, Dec 2015, Vol 496(2), pp 780–791.
- Merusi C, “Optical Diagnostics Studies of Air Flow and Powder Fluidisation In Nexthaler® – Part II: Use of Fluorescent Imaging to Characterise Transient Release of Fines From a Dry Powder Inhaler”. Int J Pharm, Oct 2018, Vol 549(1–2), pp 96–108.
- Dorosz A, Penconek A, Moskal A, “In Vitro Study on the Aerosol Emitted from the DPI Inhaler Under Two Unsteady Inhalation Profiles”. J Aerosol Sci, Nov 2016, Vol 101, pp 104–117.
- Kunda NK et al, “A Novel Approach to Study the pMDI Plume Using an Infrared Camera and to Evaluate the Aerodynamic Properties After Varying the Time Between Actuations”. Int J Pharm, Vol 526(1–2), pp 41–49.
- Chen Y et al, “High-Speed Laser Image Analysis of Plume Angles for Pressurised Metered Dose Inhalers: The Effect of Nozzle Geometry”. AAPS PharmSciTech, Apr 2017, Vol 18, pp 782–789.
- Mason-Smith N et al, “Revealing pMDI Spray Initial Conditions: Flashing, Atomisation and the Effect of Ethanol”. Pharm Res, Apr 2017, Vol 34, pp 718–729.
- Kou X et al, “Powder Dispersion Mechanisms Within a Dry Powder Inhaler Using Microscale Particle Image Velocimetry”. Int J Pharm, Dec 2016, Vol 514(2), pp 445–455.
- Ponzini R et al, “Coupled CFD-DEM Model for Dry Powder Inhalers Simulation: Validation and Sensitivity Analysis for The Main Model Parameters”. Powder Technol, Jun 2021, Vol 385, pp 199–226.
- Sommerfeld M, Cui Y, Schmalfuß S, “Potential and Constraints for the Application of CFD Combined with Lagrangian Particle Tracking to Dry Powder Inhalers”. Eur J Pharm Sci, Feb 2019, Vol 128, pp 299–324.
- Ruzycki CA, Javaheri E, Finlay WH, “The Use of Computational Fluid Dynamics in Inhaler Design”. Expert Opin Drug Deliv, Mar 2013, Vol 10(3), pp 307–323.
- Wong W et al, “The Use of Computational Approaches in Inhaler Development”. Adv Drug Deliv Rev, Mar 2012, Vol 64(4), pp 312–322.
- Suwandecha T, Wongpoowarak W, Srichana T, “Computer-Aided Design of Dry Powder Inhalers Using Computational Fluid Dynamics to Assess Performance”. Pharm Dev Technol, 2016, Vol 21(6), pp 54–60.
- Bass K, Farkas D, Longest PW, “Optimizing Aerosolization Using Computational Fluid Dynamics in a Pediatric Air-Jet Dry Powder Inhaler”. AAPS PharmSciTech, Nov 2019, Vol 20, Article 329.
- Alfano FO et al, “Full-Scale DEM Simulation of Coupled Fluid and Dry-Coated Particle Flow in Swirl-Based Dry Powder Inhalers”. Ind Eng Chem Res, Oct 2021, Vol 42, pp 15310–15326.
- Nguyen D et al, “Towards Quantitative Prediction of The Performance of Dry Powder Inhalers by Multi-Scale Simulations and Experiments”. Int J Pharm, Aug 2018, Vol 547(1–2), pp 31–43.
- Finlay W, “The Mechanics of Inhaled Pharmaceutical Aerosols – An Introduction”. Academic Press, 2019, 2nd Edition.
- Feng Y, Xu Z, Haghnegahdar A, “Computational Fluid-Particle Dynamics Modeling for Unconventional Inhaled Aerosols in Human Respiratory Systems” in “Aerosols – Science and Case Studies” (Volkov K, ed). IntechOpen, 2016.
- Tu J, Inthavong K, Ahmadi G, “Computational Fluid and Particle Dynamics in the Human Respiratory System”. Springer, 2013.
- archioli C, “Large-Eddy Simulation of Turbulent Dispersed Flows: A Review of Modelling Approaches”. Acta Mechanica, Feb 2017, Vol 228, pp 741–771.
- Mofakham AA, Ahmadi G, “On Random Walk Models for Simulation of Particle-Laden Turbulent Flows”. Int J Multiph Flow, Jan 2020, Vol 122, Article 103157.
- Gavtash B et al, “Transient Flashing Propellant Flow Models to Predict Internal Flow Characteristics, Spray Velocity, and Aerosol Droplet Size of a pMDI”. Aerosol Sci Technol, 2017, Vol 51(5), pp 564–575.
- Gavtash B et al, “Transient Aerodynamic Atomization Model to Predict Aerosol Droplet Size of Pressurized Metered Dose Inhalers”. Aerosol Sci Technol, 2017, Vol 51(8), 998–1008.
- “Skeptical About Using CFD Modeling and Simulation in the Medical and Pharmaceutical Fields?” YouTube, Maya HTT, Mar 2021.
- Kannan R et al, “Pharmaceutical Aerosols Deposition Patterns from a Dry Powder Inhaler: Euler Lagrangian Prediction and Validation”. Med Eng Phys, Apr 2017, Vol 42, pp 35–47.
- Islam MS et al, “A Review of Respiratory Anatomical Development, Air Flow Characterization and Particle Deposition”. Int J Environ Res Public Health, Jan 2020, Vol 17(2), Article 380.
- Lizal F et al, “A method for In Vitro Regional Aerosol Deposition Measurement in a Model of the Human Tracheobronchial Tree by the Positron Emission Tomography”. Proc Inst Mech Eng H, Oct 2015, Vol 229(10), pp 750–757.
- Banko AJ et al, “Three-Dimensional Inspiratory Flow in The Upper and Central Human Airways”. Exp Fluids, May 2015, Vol 56, Article 117.
- Weibel ER, Gomez DM, “Architecture of the Human Lung”. Science, Aug 1962, Vol 137(3530), pp 577–585.
- Kitaoka H, Takaki R, Suki B, “A Three-Dimensional Model of The Human Airway Tree”. J Appl Physiol, Dec 1999, Vol 87(6), pp 2207–2217.
- Koblinger L, Hofmann W, “Analysis of Human Lung Morphometric Data for Stochastic Aerosol Deposition Calculations”. Phys Med Biol, Jun 1985, Vol 30(6), pp 541–556.
- van de Moortele T, Wendt CH, Coletti F, “Morphological and Functional Properties of the Conducting Human Airways Investigated by In Vivo Computed Tomography and In Vitro MRI”. J Appl Physiol, Feb 2018, Vol 124(2), pp 400–413.
- Tian G et al, “Validating CFD Predictions of Pharmaceutical Aerosol Deposition with In Vivo Data”. Pharm Res, May 2015, Vol 32, pp 3170–3187.
- Sonnenberg AH et al, “A Markov Chain Model of Particle Deposition in the Lung”. Scientific Reports, Aug 2020, Vol 10, Article 13573.
- Koullapis P et al, “Regional Aerosol Deposition in The Human Airways: The Siminhale Benchmark Case and a Critical Assessment of In Silico Methods”. Eur J Pharm Sci, Feb 2018, Vol 113, pp 77–94.
- Zhao J, Feng Y, Fromen CA, “Glottis Motion Effects on the Particle Transport and Deposition in a Subject-Specific Mouth-To- Trachea Model: A CFPD Study”. Comput Biol Med, Jan 2020, Vol 116, Article 103532.
- Feng Y et al, “Tutorial: Understanding the Transport, Deposition, and Translocation of Particles in Human Respiratory Systems Using Computational Fluid-Particle Dynamics and Physiologically Based Toxicokinetic Models”. J Aerosol Sci, Jan 2021, Vol 151, Article 105672.
- Sommerfeld M, van Wachem B, Oliemans R, “Computational Fluid Dynamics of Dispersed Multi-Phase Flows”. ERCOFTAC Best Practice Guidelines, 2008.
- Koullapis P et al, “Aerosol Deposition in the Human Upper Airways”. ERCOFTAC Best Practice Guidelines, 2019.