Citation: Gertz F, Steffen Y, “Data-Driven Sustainability in Autoinjector Development”. ONdrugDelivery, Issue 126 (Oct/Nov 2021), pp 52–56.
Frederick Gertz and Yves Steffen outline SHL Medical’s approach to sustainability, involving leveraging the experience of its data science department to enhance the output of its sustainability department.
“SHL has derived an interesting partnership between its data-science efforts and its sustainability efforts.”
What does it truly mean to be sustainable and how can we achieve it? SHL Medical is not alone in asking these questions. Going beyond the obvious reduction in carbon footprints, sustainability encompasses a whole multifaceted paradigm for operating a company. If the UN Global Compact Principles are used as a guideline, it encompasses climate, social justice, education, health and equality, to name just a few areas.1 For SHL, the goal remains the same – how to produce products in such a way that leaves the world a better place, for the planet and its people. To that end, SHL has found the best way to be more sustainable is to keep its footprint small to begin with.
Over the decades, SHL has made great efforts to increase its efficiency, to incorporate lean thinking and implementations into its production and supply, and to leverage the most cutting-edge automation and information technologies. This continuous improvement process has shown that sustainability is efficiency – and the company’s goals are not hindered by its aim to be a sustainable company.
In this holistic approach to sustainability, SHL has derived an interesting partnership between its data-science efforts and its sustainability efforts. Since one of the goals of the data-science department is to enhance internal processes and improve efficiency, SHL has begun marrying the efforts of the two areas to bring new insight to its sustainability initiatives while also helping it to maintain its leading position as a solutions provider of autoinjector devices. These efforts have helped expand the company’s data-driven decision processes beyond pure business decisions. SHL is uniquely positioned to provide this sort of value due to its vertically integrated structure and 30 years of experience in the field.
“Sustainability initiatives and thinking in the organisation help SHL by providing it with additional robust parameters on which it can optimise.”
THE CONVERGENCE OF DATA AND SUSTAINABILITY
So how does the data-driven portion of an operation meld with the sustainability areas? The answer is best found in both past innovations for process development and also in future areas. Many companies today focus their control methodology on statistical principles, with the Six Sigma approach being the most commonly observed within the industry. The techniques aim to improve quality by limiting variation within the process so that a consistent product is almost always produced. Six Sigma techniques for control strategies came from Japan in the 1970s and were popularised by car manufacturer Toyota. In that same era, another Japanese school of quality was also being developed by Genichi Taguchi. His proposed system for quality incorporated loss functions as a sort of mathematical description of the impact that each measured characteristic would have on a certain outcome.
In brief, loss function refers to a mathematical description of a preferred outcome using the data currently generated in the company to quantify how far away the current situation is from the ideal. What is interesting about this methodology is that much of the debate around it is centred around the discussion of what the loss function should maximise. For most corporations, the answer is usually either quality or revenue or something similar. Mr Taguchi thought otherwise and believed that “social good” was to be maximised. Built into the mathematical function that Mr Taguchi described, known as the “Taguchi loss function”, were variables associated with social wellness and benefit.
“The use of sustainable materials and techniques can even be a source of innovation.”
The data-science team has begun developing ways to incorporate this aspect of thinking into SHL’s own control plans and quality improvement initiatives, with optimisations focused not just around typical quality outputs but also incorporating aspects of energy efficiency and human efficiency. In fact, much of this has led the way for research in using data science in production environments. Already, companies have used the large data outputs from their internal manufacturing execution systems to derive areas of increased energy efficiency. In the manufacturing of moulded plastics in particular, where the entire plant essentially operates as a large heat exchanger,2 there are numerous opportunities to improve cycle times, energy use and effective heat transfer to lower carbon footprints. Similarly, these areas are further enhanced when they are leveraged to reduce scrap rates, ensuring that as little material as possible is wasted throughout the production process.
These areas of optimisation may seem familiar – and that is because they are. Energy efficiency, scrap rate and higher throughput are all areas that any company would strive towards to improve their production output during their normal continuous improvement process. The areas also highlight how these sustainability efforts are in no way removed from the typical operations of any modern manufacturing operation. Sustainability issues can in some corporations be seen as a burden, an additional obstacle that must be overcome, but SHL has seen that these endeavours are in fact an additional pathway to success.
Sustainability initiatives and thinking in the organisation help SHL by providing it with additional robust parameters on which it can optimise. This robustness can give additional performance indicators to maximise, and further help highlight how production improvement efforts are having effects beyond just the financial bottom line. The additional parameter for project success makes some decisions easier. For example, if two options seem essentially equal, the company can now select the one that has the greatest effect on its sustainability goals. These types of avenues and endeavours can be a great enhancement to any company’s data-driven efforts, and SHL continues to embrace these techniques.
AI-ENHANCED SUSTAINABILITY INNOVATION
Factory-based artificial intelligence (AI) implementations have also shown a large performance increase over standard techniques, particularly in areas where automated controls are being used. The use of AI within the tool chain of programmable logic controls can allow for drastic increases in efficiency (Figure 1). A good example of this is in the control of asynchronous motors, where machine-learning techniques from neural networks to genetic algorithms have been used, and shown to consistently outperform standard controllers.3 For many operations, this is incredibly exciting as the intuition of many factory managers is that the switch to electric-based motors had already drastically increased performance, and they are under the impression that further increases in efficiency are just not available to them.
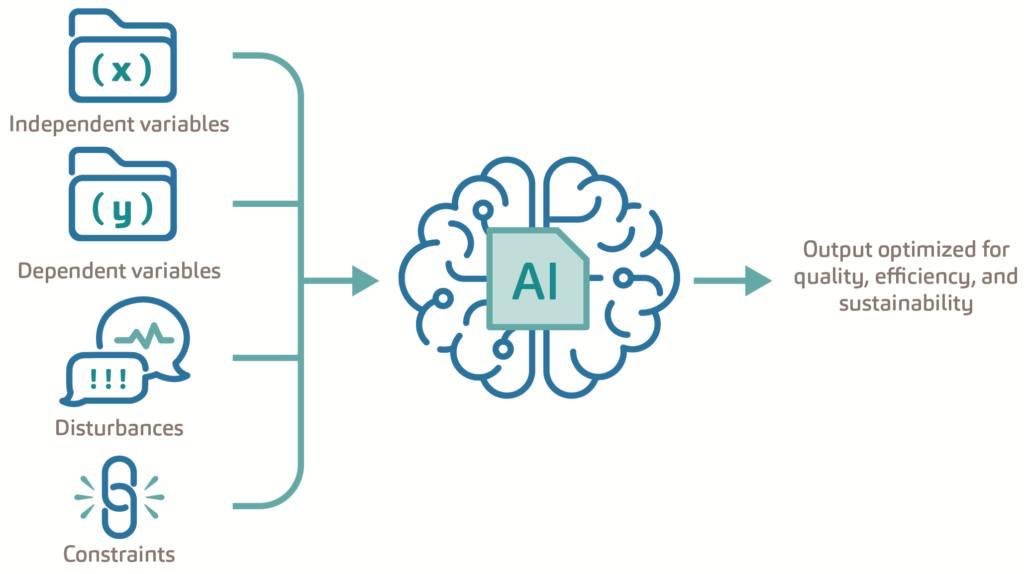
Figure 1: Diagram depicting how AI can use large amounts of data to produce value from a variety of disparate sources.
Further, the use of sustainable materials and techniques can even be a source of innovation. By using generative methods and a variety of other cutting-edge techniques, much research has investigated the use of AI to help solve design problems. A better-known use of these solutions has been in the area of materials science, where high-dimensional materials spaces can be defined using a variety of different input factors. These high-dimensional spaces are well suited for solutions from new AI techniques, such as attention-based networks,4 and can allow companies to iterate on many materials to find one that is not only best suited for their design but also well suited for their sustainability goals. In this way, companies might explore multiple alternatives that they might not have originally considered, allowing for innovative use of novel materials.
“It is easy to say that you desire to have your entire factory run more efficiently but, in a practical sense, you will need to choose your battles.”
TWO SIDES OF THE SAME COIN
In the interest of corporate and global welfare, SHL is keen to encourage others to adopt its so-called data-driven approach to sustainability. But once you combine the efforts of these two, now seemingly well-aligned teams together, the next step is how best to leverage them to have real world impact.
One of the first goals of any such marriage is to work together to define success. Your sustainability team, depending on their maturity, will either have predefined metrics for success or will be currently forming them. In the latter case, the data science team can play an important part in providing reasonable goals – ones that truly help the initiative as well as ones that are reasonably obtainable. Once the agreed-upon metrics have been determined, whether it be related to waste, energy use or carbon footprint, to name but a few, the data-science team can begin to work on helping prioritise your goals. Using energy savings as an example, it is easy to say that you desire to have your entire factory run more efficiently but, in a practical sense, you will need to choose your battles.
Here is where data science is truly beneficial. By combining information across your entire manufacturing space and using cost functions related to your sustainability goals, your team can begin to optimise and, most importantly, prioritise which areas of the operation not only use the most energy but also are most capable of being changed to a more efficient status. Using this data, as well as real-time analytics, the sustainability team can immediately begin selecting areas with the highest impact and visualise those changes to highlight their benefits.
It is also important to highlight that choosing these goals in no way must be misaligned with business goals. Using well-developed methods from operations research, and understanding the company’s current financial models and projections, the team can also optimise to have the smallest impact on those plans – and even find areas to enhance some of those opportunities. Most companies will find that about 1–2% of their bottom lines5 get attributed to environmental areas. But with this complete data model, those numbers can be significantly enhanced. Furthermore, this cross-departmental look, with strong analytics and improvements underlying the efforts, can also highlight something truly important for the sustainability team − its ability to enhance the revenue of the company through its objectives.
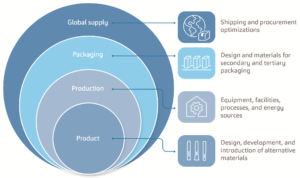
Figure 2: A visual depiction of the highly dependent nature of autoinjector development from design to production to delivery.
SHL’S SUSTAINABILITY GOALS
Former Unilever Chief Executive Officer Paul Polman once said: “We cannot choose between [economic] growth and sustainability – we must have both.”6 To this end, SHL has endeavoured to create “win-win” scenarios where it is able, through its data-driven methodology, to find areas where improvements in sustainable growth also improve the company’s market competitiveness. It is implementing innovative initiatives across its portfolio and sites, with the goal of making all its products inherently sustainable. These sustainability initiatives cover not just the product design, development and production streams but also secondary and tertiary packaging, as well as other supply chain activities (Figure 2).
SHL continues to strive to be not just a leader in autoinjectors but also a leader in sustainable manufacturing across a variety of different sustainability targets. The company considers its combination approach – leveraging the experience of its data-science department to enhance the output of its sustainability department – as a novel offering and it hopes its experience helps highlight the possibilities for other teams looking to provide a production environment that ultimately serves both company goals and also shared objectives for global social responsibility.
REFERENCES
- Vinuesa R et al, “The role of artificial intelligence in achieving the Sustainable Development Goals”. Nature Communications, 2020, Vol 11, pp 1–10.
- Song B et al, “Data-driven Approach for Discovery of Energy Saving Potentials in Manufacturing Factory”. Procedia CIRP, 2018, Vol 69, pp 330–335.
- Rousopoulou V et al, “Predictive Maintenance for Injection Molding Machines Enabled by Cognitive Analytics for Industry 4.0”. Front Artif Intell, 2020.
- Dunn J (Ed), Balaprakash P (Ed), “Data Science Applied to Sustainability Analysis”. Elsevier, May 11, 2021. (ISBN: 9780128179765)
- Taylor T, “The Instant Economist: Everything You Need to Know About How the Economy Works”. Plume (Penguin Group), Jan 31, 2012. (ISBN: 9780452297524)
- Pickering A, “Purpose After Polman: How the Poster Child for Purpose Paved the Way for Future Leadership”. Sustainable Brands, Online, Dec 24, 2017. (Accessed October 2021)